Introduction
The key to getting better at deep learning (or most fields in life) is practice. Practice on a variety of problems – from image processing to speech recognition. Each of these problem has it’s own unique nuance and approach.
But where can you get this data? A lot of research papers you see these days use proprietary datasets that are usually not released to the general public. This becomes a problem, if you want to learn and apply your newly acquired skills.
If you have faced this problem, we have a solution for you. We have curated a list of openly available datasets for your perusal.
In this article, we have listed a collection of high quality datasets that every deep learning enthusiast should work on to apply and improve their skillset. Working on these datasets will make you a better data scientist and the amount of learning you will have will be invaluable in your career. We have also included papers with state-of-the-art (SOTA) results for you to go through and improve your models.
How to use these datasets?
First things first – these datasets are huge in size! So make sure you have a fast internet connection with no / very high limit on the amount of data you can download.
There are numerous ways how you can use these datasets. You can use them to apply various Deep Learning techniques. You can use them to hone your skills, understand how to identify and structure each problem, think of unique use cases and publish your findings for everyone to see!
The datasets are divided into three categories – Image Processing, Natural Language Processing, and Audio/Speech Processing.
Let’s dive into it!
Image Datasets
MNIST
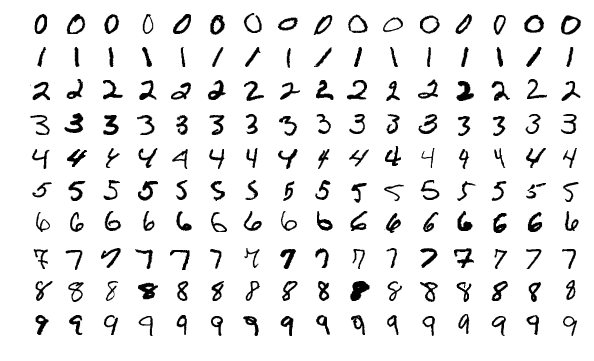
MNIST is one of the most popular deep learning datasets out there. It’s a dataset of handwritten digits and contains a training set of 60,000 examples and a test set of 10,000 examples. It’s a good database for trying learning techniques and deep recognition patterns on real-world data while spending minimum time and effort in data preprocessing.
Size: ~50 MB
Number of Records: 70,000 images in 10 classes
MS-COCO
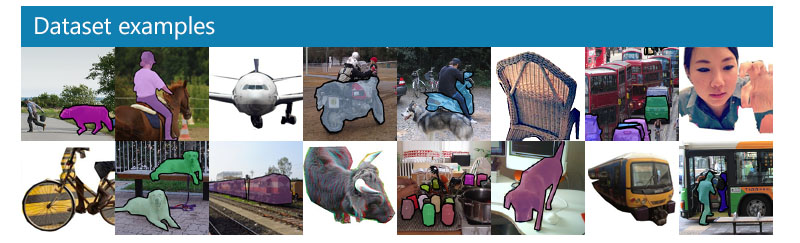
COCO is a large-scale and rich for object detection, segmentation and captioning dataset. It has several features:
- Object segmentation
- Recognition in context
- Superpixel stuff segmentation
- 330K images (>200K labeled)
- 1.5 million object instances
- 80 object categories
- 91 stuff categories
- 5 captions per image
- 250,000 people with keypoints
Size: ~25 GB (Compressed)
Number of Records: 330K images, 80 object categories, 5 captions per image, 250,000 people with key points
SOTA : Mask R-CNN
ImageNet

ImageNet is a dataset of images that are organized according to the WordNet hierarchy. WordNet contains approximately 100,000 phrases and ImageNet has provided around 1000 images on average to illustrate each phrase.
Size: ~150GB
Number of Records: Total number of images: ~1,500,000; each with multiple bounding boxes and respective class labels
Open Images Dataset
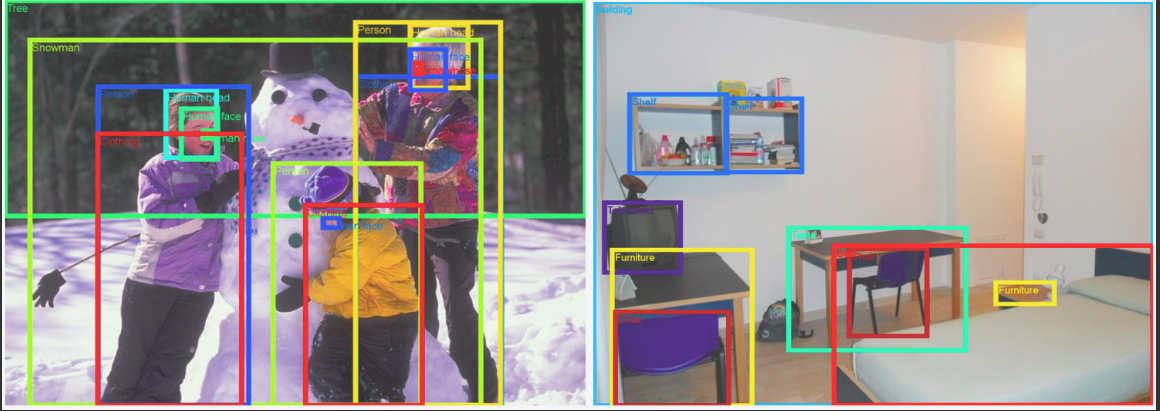
Open Images is a dataset of almost 9 million URLs for images. These images have been annotated with image-level labels bounding boxes spanning thousands of classes. The dataset contains a training set of 9,011,219 images, a validation set of 41,260 images and a test set of 125,436 images.
Size: 500 GB (Compressed)
Number of Records: 9,011,219 images with more than 5k labels
SOTA : Resnet 101 image classification model (trained on V2 data): Model checkpoint, Checkpoint readme, Inference code.
VisualQA
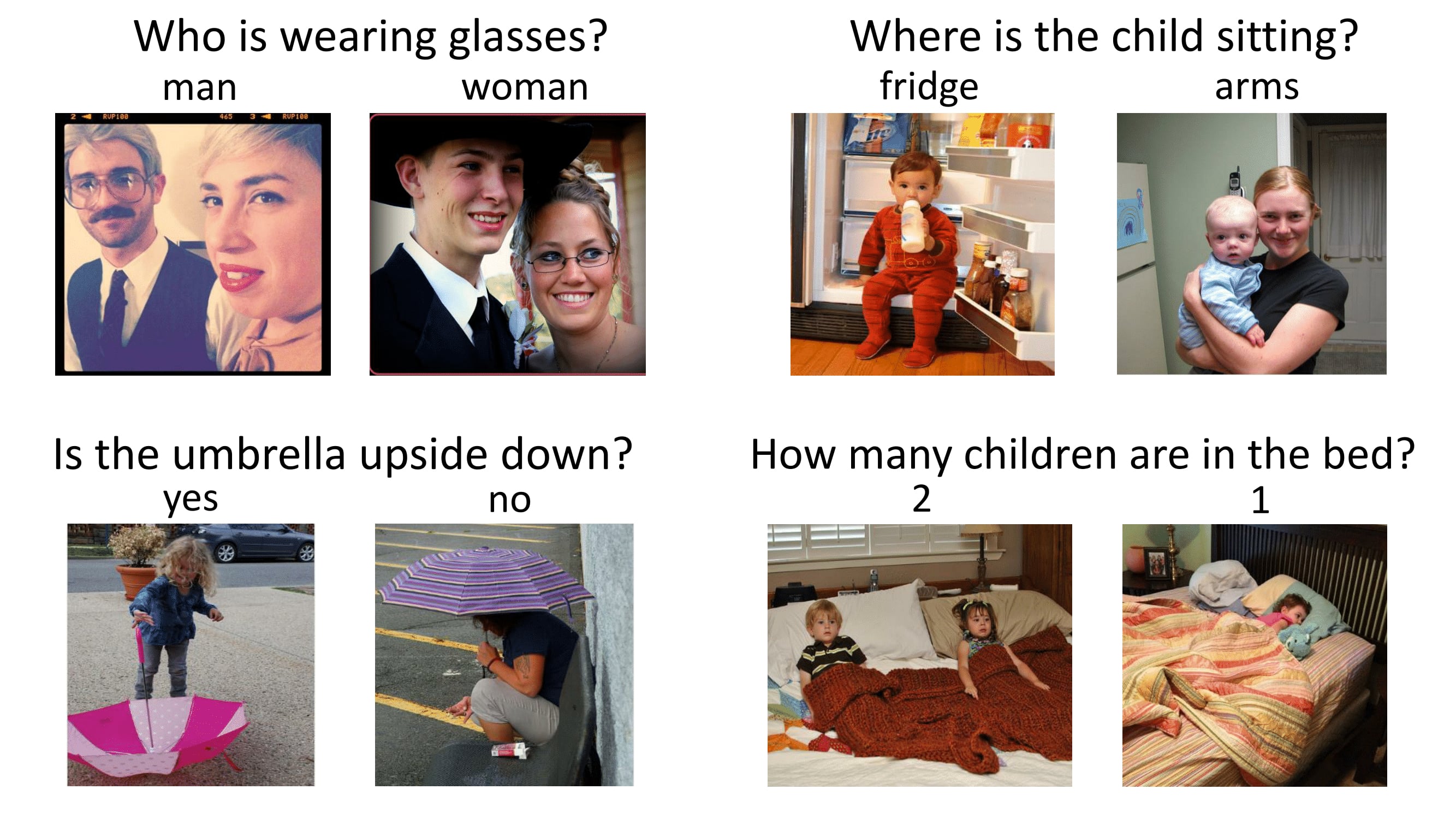
VQA is a dataset containing open-ended questions about images. These questions require an understanding of vision and language. Some of the interesting features of this dataset are:
- 265,016 images (COCO and abstract scenes)
- At least 3 questions (5.4 questions on average) per image
- 10 ground truth answers per question
- 3 plausible (but likely incorrect) answers per question
- Automatic evaluation metric
Size: 25 GB (Compressed)
Number of Records: 265,016 images, at least 3 questions per image, 10 ground truth answers per question
The Street View House Numbers (SVHN)
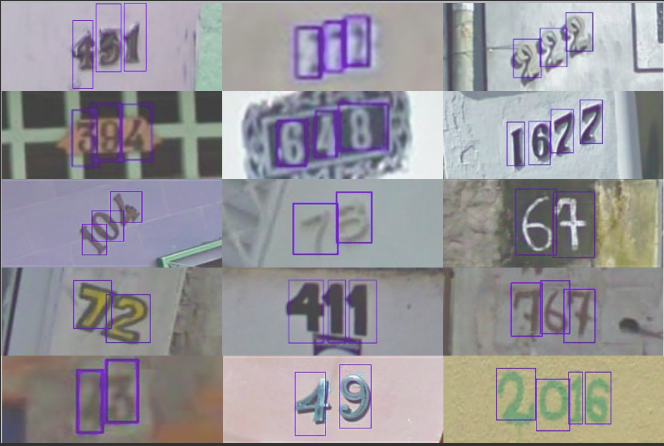
This is a real-world image dataset for developing object detection algorithms. This requires minimum data preprocessing. It is similar to the MNIST dataset mentioned in this list, but has more labelled data (over 600,000 images). The data has been collected from house numbers viewed in Google Street View.
Size: 2.5 GB
Number of Records: 6,30,420 images in 10 classes
CIFAR-10
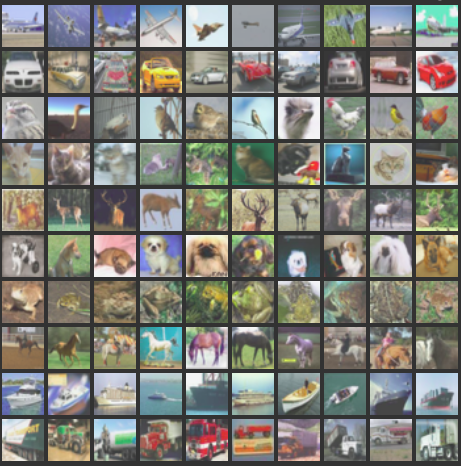
This dataset is another one for image classification. It consists of 60,000 images of 10 classes (each class is represented as a row in the above image). In total, there are 50,000 training images and 10,000 test images. The dataset is divided into 6 parts – 5 training batches and 1 test batch. Each batch has 10,000 images.
Size: 170 MB
Number of Records: 60,000 images in 10 classes
SOTA : ShakeDrop regularization
Fashion-MNIST
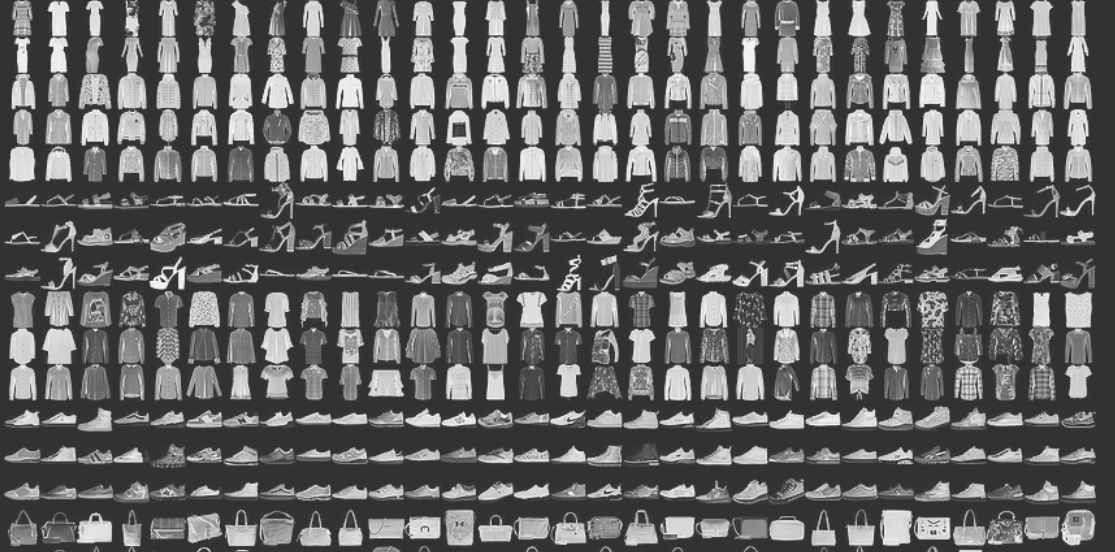
Fashion-MNIST consists of 60,000 training images and 10,000 test images. It is a MNIST-like fashion product database. The developers believe MNIST has been overused so they created this as a direct replacement for that dataset. Each image is in greyscale and associated with a label from 10 classes.
Size: 30 MB
Number of Records: 70,000 images in 10 classes
Natural Language Processing
IMDB Reviews
This is a dream dataset for movie lovers. It is meant for binary sentiment classification and has far more data than any previous datasets in this field. Apart from the training and test review examples, there is further unlabeled data for use as well. Raw text and preprocessed bag of words formats have also been included.
Size: 80 MB
Number of Records: 25,000 highly polar movie reviews for training, and 25,000 for testing
Twenty Newsgroups
This dataset, as the name suggests, contains information about newsgroups. To curate this dataset, 1000 Usenet articles were taken from 20 different newsgroups. The articles have typical features like subject lines, signatures, and quotes.
Size: 20 MB
Number of Records: 20,000 messages taken from 20 newsgroups
Sentiment140
Sentiment140 is a dataset that can be used for sentiment analysis. A popular dataset, it is perfect to start off your NLP journey. Emotions have been pre-removed from the data. The final dataset has the below 6 features:
- polarity of the tweet
- id of the tweet
- date of the tweet
- the query
- username of the tweeter
- text of the tweet
Size: 80 MB (Compressed)
Number of Records: 1,60,000 tweets
WordNet
Mentioned in the ImageNet dataset above, WordNet is a large database of English synsets. Synsets are groups of synonyms that each describe a different concept. WordNet’s structure makes it a very useful tool for NLP.
Size: 10 MB
Number of Records: 117,000 synsets is linked to other synsets by means of a small number of “conceptual relations.
Yelp Reviews
This is an open dataset released by Yelp for learning purposes. It consists of millions of user reviews, businesses attributes and over 200,000 pictures from multiple metropolitan areas. This is a very commonly used dataset for NLP challenges globally.
Size: 2.66 GB JSON, 2.9 GB SQL and 7.5 GB Photos (all compressed)
Number of Records: 5,200,000 reviews, 174,000 business attributes, 200,000 pictures and 11 metropolitan areas
SOTA : Attentive Convolution
The Wikipedia Corpus
This dataset is a collection of a the full text on Wikipedia. It contains almost 1.9 billion words from more than 4 million articles. What makes this a powerful NLP dataset is that you search by word, phrase or part of a paragraph itself.
Size: 20 MB
Number of Records: 4,400,000 articles containing 1.9 billion words
The Blog Authorship Corpus
This dataset consists of blog posts collected from thousands of bloggers and has been gathered from blogger.com. Each blog is provided as a separate file. Each blog contains a minimum of 200 occurrences of commonly used English words.
Size: 300 MB
Number of Records: 681,288 posts with over 140 million words
Machine Translation of Various Languages
This dataset consists of training data for four European languages. The task here is to improve the current translation methods. You can participate in any of the following language pairs:
- English-Chinese and Chinese-English
- English-Czech and Czech-English
- English-Estonian and Estonian-English
- English-Finnish and Finnish-English
- English-German and German-English
- English-Kazakh and Kazakh-English
- English-Russian and Russian-English
- English-Turkish and Turkish-English
Size: ~15 GB
Number of Records: ~30,000,000 sentences and their translations
SOTA : Attention Is All You Need
Audio/Speech Datasets
Free Spoken Digit Dataset
Another entry in this list for inspired by the MNIST dataset! This one was created to solve the task of identifying spoken digits in audio samples. It’s an open dataset so the hope is that it will keep growing as people keep contributing more samples. Currently, it contains the below characteristics:
- 3 speakers
- 1,500 recordings (50 of each digit per speaker)
- English pronunciations
Size: 10 MB
Number of Records: 1,500 audio samples
Free Music Archive (FMA)
FMA is a dataset for music analysis. The dataset consists of full-length and HQ audio, pre-computed features, and track and user-level metadata. It an an open dataset created for evaluating several tasks in MIR. Below is the list of csv files the dataset has along with what they include:
tracks.csv
: per track metadata such as ID, title, artist, genres, tags and play counts, for all 106,574 tracks.genres.csv
: all 163 genre IDs with their name and parent (used to infer the genre hierarchy and top-level genres).features.csv
: common features extracted with librosa.echonest.csv
: audio features provided by Echonest (now Spotify) for a subset of 13,129 tracks.
Size: ~1000 GB
Number of Records: ~100,000 tracks
Ballroom
This dataset contains ballroom dancing audio files. A few characteristic excerpts of many dance styles are provided in real audio format. Below are a few characteristics of the dataset:
- Total number of instances: 698
- Duration: ~30 s
- Total duration: ~20940 s
Size: 14GB (Compressed)
Number of Records: ~700 audio samples
Million Song Dataset
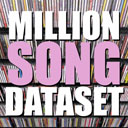
The Million Song Dataset is a freely-available collection of audio features and metadata for a million contemporary popular music tracks. Its purposes are:
- To encourage research on algorithms that scale to commercial sizes
- To provide a reference dataset for evaluating research
- As a shortcut alternative to creating a large dataset with APIs (e.g. The Echo Nest’s)
- To help new researchers get started in the MIR field
The core of the dataset is the feature analysis and metadata for one million songs. The dataset does not include any audio, only the derived features. The sample audio can be fetched from services like7digital, using code provided by Columbia University.
Size: 280 GB
Number of Records: PS – its a million songs!
LibriSpeech
This dataset is a large-scale corpus of around 1000 hours of English speech. The data has been sourced from audiobooks from the LibriVox project. It has been segmented and aligned properly. If you’re looking for a starting point, check out already prepared Acoustic models that are trained on this data set at kaldi-asr.org and language models, suitable for evaluation, at http://www.openslr.org/11/.
Size: ~60 GB
Number of Records: 1000 hours of speech
VoxCeleb
VoxCeleb is a large-scale speaker identification dataset. It contains around 100,000 utterances by 1,251 celebrities, extracted from YouTube videos. The data is mostly gender balanced (males comprise of 55%). The celebrities span a diverse range of accents, professions and age. There is no overlap between the development and test sets. It’s an intriguing use case for isolating and identifying which superstar the voice belongs to.
Size: 150 MB
Number of Records: 100,000 utterances by 1,251 celebrities
Analytics Vidhya Practice Problems
For your practice, we also provide real life problems and datasets to get your hands dirty. In this section, we’ve listed down the deep learning practice problems on our DataHack platform.
Twitter Sentiment Analysis
Hate Speech in the form of racism and sexism has become a nuisance on twitter and it is important to segregate these sort of tweets from the rest. In this Practice problem, we provide Twitter data that has both normal and hate tweets. Your task as a Data Scientist is to identify the tweets which are hate tweets and which are not.
Size: 3 MB
Number of Records: 31,962 tweets
Age Detection of Indian Actors
This is a fascinating challenge for any deep learning enthusiast. The dataset contains thousands of images of Indian actors and your task is to identify their age. All the images are manually selected and cropped from the video frames resulting in a high degree of variability interms of scale, pose, expression, illumination, age, resolution, occlusion, and makeup.
Size: 48 MB (Compressed)
Number of Records: 19,906 images in the training set and 6636 in the test set
Urban Sound Classification
This dataset consists of more than 8000 sound excerpts of urban sounds from 10 classes. This practice problem is meant to introduce you to audio processing in the usual classification scenario.
Size: Training set – 3 GB (Compressed), Test set – 2 GB (Compressed)
Number of Records: 8732 labeled sound excerpts (<=4s) of urban sounds from 10 classes